Curating the collected data
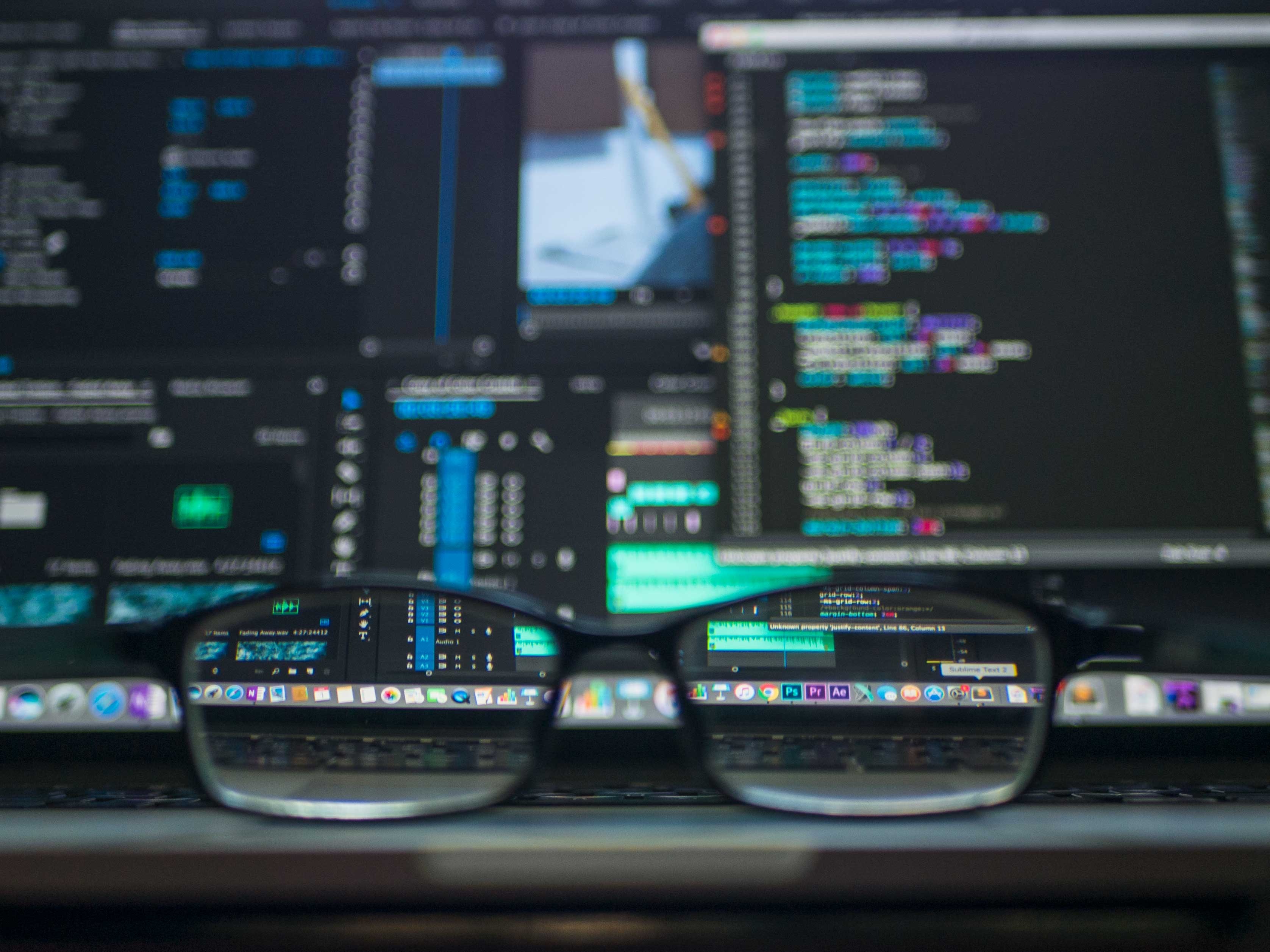
Following the collection of data from the first study of the POSTCOVID-AI project, the team has embarked on a meticulous and necessary task: data curation. The data collected is heterogeneous in nature, i.e. diverse. They range from sensor data, such as continuous numerical records of brightness or environmental noise measurements, to categorical values of physical activity or textual responses to some of the surveys. All these records are susceptible to reflecting values outside the permitted ranges, duplicate records or even the absence or loss of some of them. This is why it is necessary to curate or harmonise them in order to generate a clean and functional database.
Although there are several curation techniques, most of them coming from the so-called Data Science field, the main methods used in our project are labelling, coding, deletion of non-relevant fields, elimination of outliers, and imputation of missing values. Labelling and coding allow the contextualisation of some of the recorded values so that the user of the data can clearly understand what they refer to (for example, indicating the physical activity performed by each user at each instant of time recorded). Field deletion allows the deletion of records that are technically necessary but do not add value to the dataset (e.g. the time at which the data was sent from the mobile application to the server). The elimination of outliers refers to the use of statistical techniques that detect numerical values that escape the normal distribution of the values (e.g. an absurdly high environmental noise value). Finally, imputation also makes use of statistical techniques such as interpolation to fill in some of the gaps generated by the missing data record.